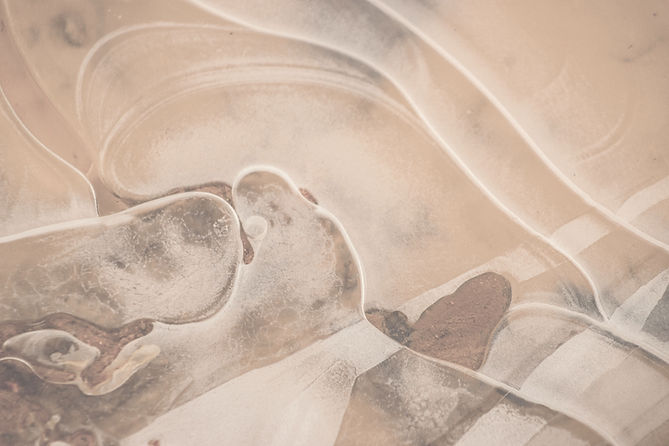
My Research
I am at the forefront of groundbreaking research that delves into the intersection of artificial intelligence, machine learning, natural language processing, and neural network technologies, with a primary focus on revolutionizing the landscape of medical and healthcare practices. My innovative approach harnesses the power of these cutting-edge technologies to develop advanced models designed for predicting, diagnosing, and treating life-threatening medical conditions with high accuracy. With a particular emphasis on ailments such as cardiovascular disease, cancer, diabetes, and diabetic retinopathy, my work seeks to usher in a new era of precision medicine. By leveraging the capabilities of AI and machine learning, my models aim to enhance medical decision-making, optimize treatment strategies, and ultimately contribute to improved patient outcomes. My research represents a significant stride towards harnessing the potential of technology to address critical health challenges, showcasing the transformative impact of integrating artificial intelligence into the medical and healthcare domain.

01
AI & ML in Cardiovascular Disease
My research revolves around developing innovative machine learning models with a primary focus on addressing critical challenges in cardiovascular diseases. The overarching goal is to enhance the survival rates of patients grappling with severe heart conditions by leveraging artificial intelligence, machine learning, neural networks, and cutting-edge technologies. The collaboration with a renowned multispecialty hospital is a key aspect of the project, emphasizing the importance of process and system automation in more effectively managing cardiovascular diseases.
The core objectives of my work include the creation of a novel healthcare machine learning framework for estimating heart chamber elasticity, devising statistical models for the early detection of cardiac arrest, congenital abnormalities, and assessing blockage severity. This multifaceted approach encompasses image analysis, neural network-based heart rate detection, and the application of deep learning and Fourier Transform Infrared Spectroscopy (FTIR) for cardiac blood vessel analysis. The research addresses existing limitations in accurately predicting early symptoms and the likelihood of cardiac arrest, spanning across different age groups and considering various factors such as lifestyle, genetics, and determinants contributing to cardiovascular issues.
The success of my project is evident in the development of advanced machine learning models and the publication of research articles in esteemed journals and conferences. The outcomes of this research hold significant potential to positively impact the prognosis of cardiac conditions, elevate survival rates, and contribute valuable insights to the broader understanding of cardiovascular diseases.
02
AI & ML in Cancer
My research is focused on developing machine learning models for the early detection and classification of leukemia, a form of blood cancer. Leukemia is a critical health concern, impacting the blood and bone marrow, and early and accurate diagnosis is pivotal for effective treatment. The project aimed to overcome existing diagnostic limitations by incorporating machine learning, deep learning, and statistical analysis to enhance the precision and efficiency of leukemia detection.
The research employed a diverse dataset comprising 10,000 images, with a specific emphasis on distinguishing leukemic cells from normal ones in peripheral blood smear (PBS) images. Challenges such as morphological similarities between healthy and leukemic cells, staining noise, and subject-level variability were addressed through advanced statistical parameters, the development of a convolutional neural network (CNN) using Python and TensorFlow, and the implementation of stain color normalization techniques. The project's outcomes, published in esteemed journals like IEEE Access, showcased a notable level of accuracy in leukemia cell classification and pattern recognition. The significance of this work lies in its potential to enhance the precision, cost-effectiveness, and speed of leukemia diagnosis, ultimately contributing to improved patient outcomes and survival rates.

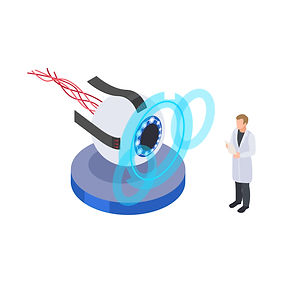
03
AI & ML in Diabetic Retinopathy
My research is focused on the implementation of machine learning in addressing crucial aspects of diabetic retinopathy (DR). Diabetic retinopathy is a significant complication arising from diabetes, leading to severe vision impairment or blindness. Recognizing the pressing need for advanced technologies to overcome challenges in existing research projects, I embarked on a comprehensive study to develop predictive models using machine learning techniques.
One key aspect of my work involved the creation of a convolutional neural network (CNN) algorithm designed to detect and classify diabetic retinopathy in clinical images of the retina. Leveraging high-resolution images from the IDRiD dataset, I trained the model using specific parameters and achieved promising results in the classification of DR and its severity stages. This contribution addresses a critical gap in the field, providing a tool for early identification and monitoring of diabetic retinopathy, crucial for timely intervention and improved patient outcomes.
Additionally, the research extended to predicting insulin dosage for diabetic patients, employing recurrent neural networks (RNN) with long short-term memory (LSTM) and artificial neural networks (ANN). By developing models that accurately predict insulin needs based on historical blood sugar levels, my work contributes to the effective management of diabetes, potentially reducing the risks and complications associated with uncontrolled blood glucose levels. Overall, this research represents a valuable interdisciplinary effort, combining machine learning techniques with a focus on real-world medical challenges in diabetes and its associated complications, particularly in the context of diabetic retinopathy.